
Unveiling the Hidden Structure of Tech Roles
8 minute read
Introduction
Technology has become integral to our daily lives as we navigate the digital era. However, keeping up with ever-changing advancements can be challenging, especially in the field of recruitment. Accurately matching candidates to specific tech roles can be daunting. To solve this issue, we have developed a unique approach to document classification. This method automates the process and uncovers the underlying structure of tech roles.
The Power of Taxonomy
Consistency is key when it comes to building a successful blog. This involves publishing content on a regular schedule. Readers will anticipate and look forward to fresh content from your blog. Failure to provide it may lead to a loss of interest.
Introducing the Multiclass Document Classifier
Our multiclass, semi-supervised document classifier is designed explicitly for tech jobs. We use cutting-edge techniques to develop an intrinsic model of the data structure while using minimal labeled data. This approach ensures accurate classification and provides explainable features, creating a taxonomy of technology roles.
Unveiling the Data Structure
Our classifier performs excellently even with limited training data, making it ideal as a standalone classifier for tech roles. With this model, you can gain insights into the underlying data structure. The resulting taxonomy visually represents the distribution and relationships of critical skills, allowing for a comprehensive understanding of tech roles.
The Process
We first preprocess the data and extract key phrases and skills. These skills are then grouped into clusters using our custom Skill Extractor, which weights them based on their relevance to the job description.
The Algorithm
Our variation of the k-Nearest Neighbors (kNN) algorithm incorporates weighted references. The algorithm generates role centroids through training and inference stages, and regards the primary skills that form the basis for classification. We can predict the most relevant roles for a given job description by comparing vectors and distances.
Great Results and Practical Applications
Our classifier achieves great accuracy even with a small labeled dataset, showing its ability to distinguish between closely related tech roles. Furthermore, we can demonstrate how the learned structure and features can be used beyond document classification; for example to generate labels for more complex classifiers, understand company taxonomies, and facilitate career transitions.
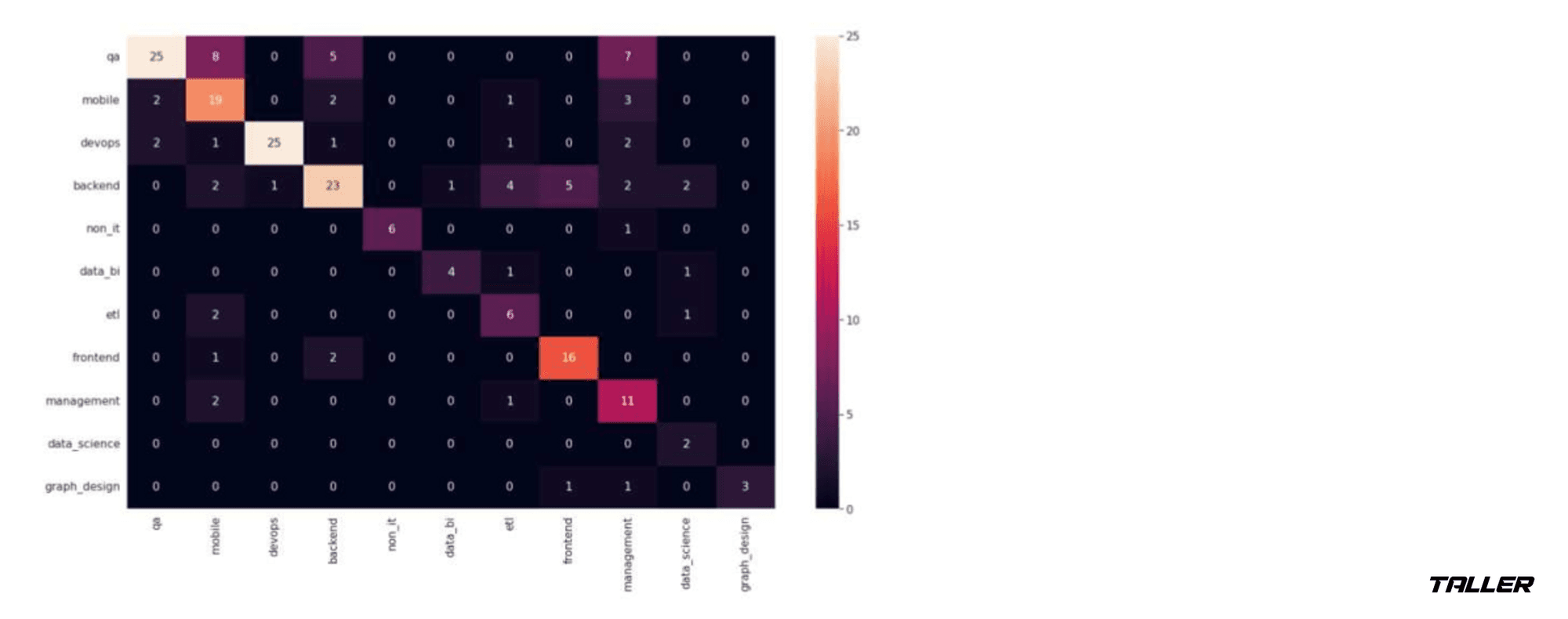
The following graphical representations show the taxonomy of two different areas within a particular company. These graphs provide a better understanding of how the company defines each area.
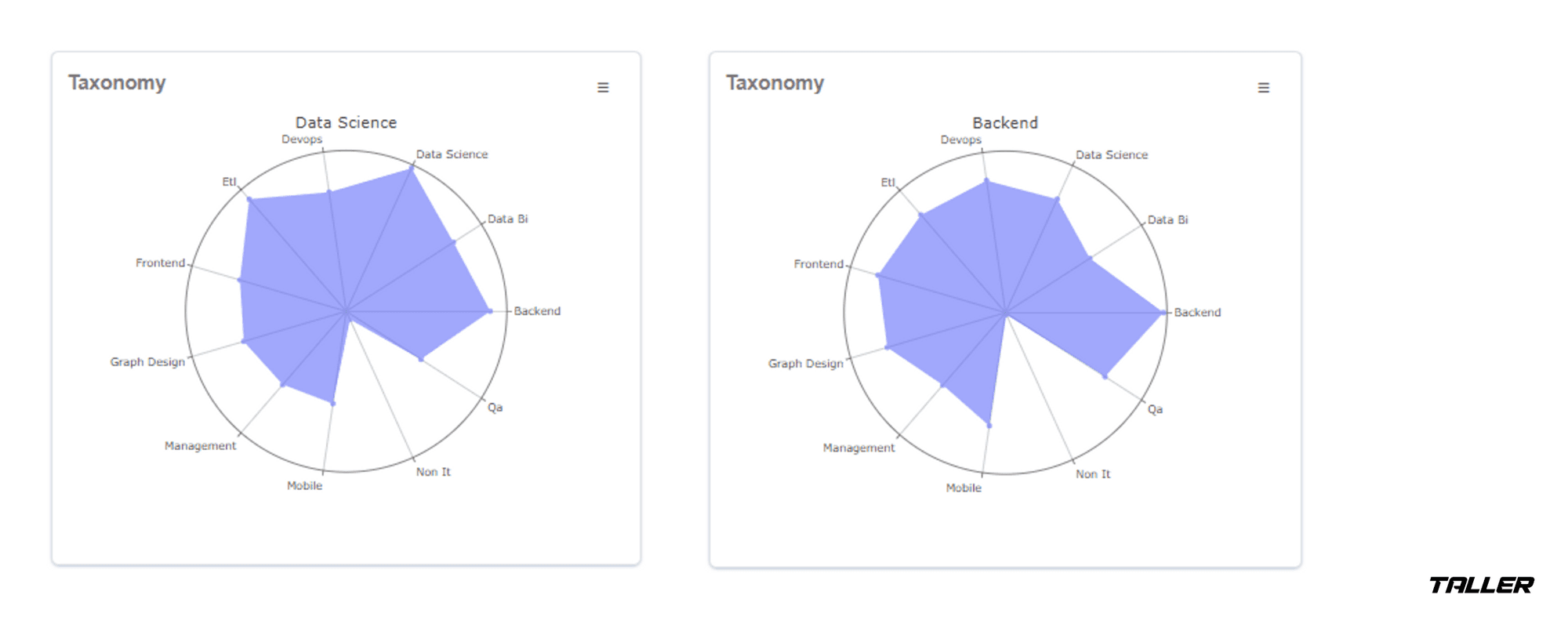
Thanks to our Multiclass Document Classifier, we were able to develop various sub-products that greatly enhance the Taller business model. These include a Relevant Skills Detector for each area, an Automatic Tech Stacks Detector, and more. These products are generated automatically using a company’s publicly available information.
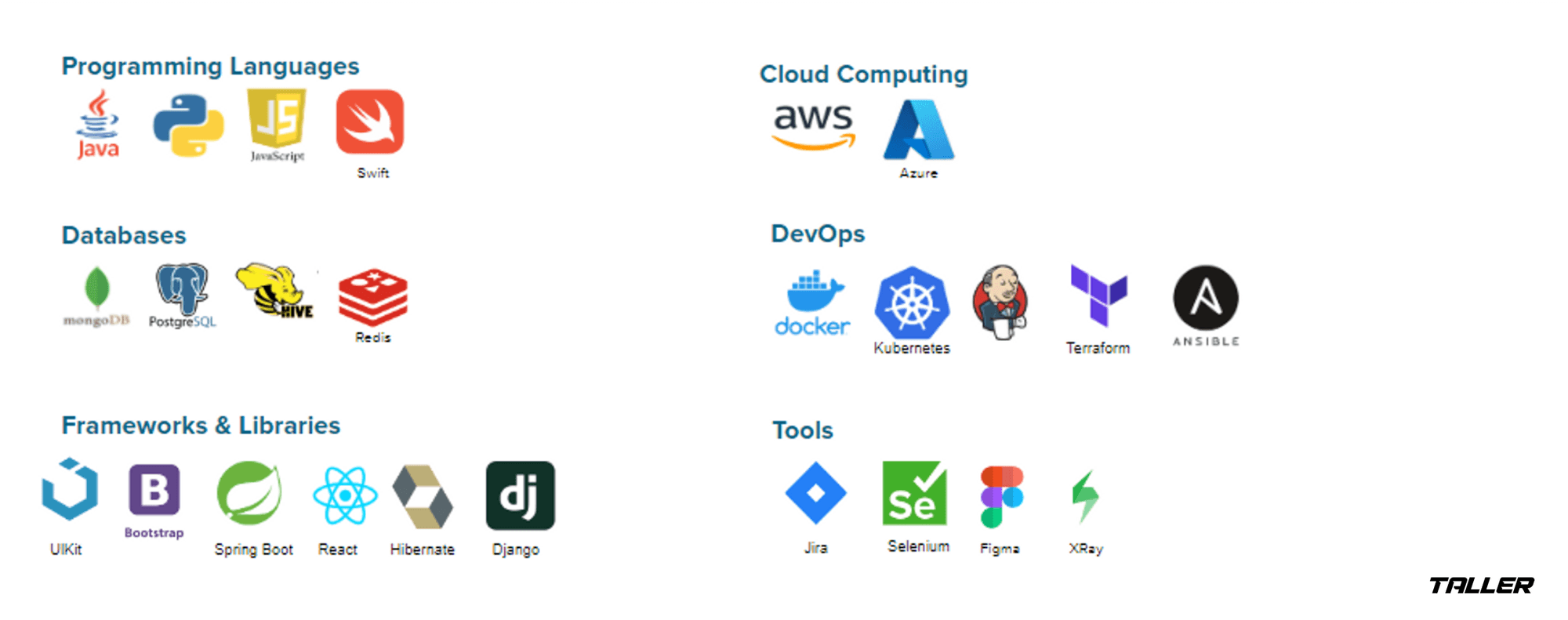
Given its abilities, this revolutionary tool has the potential to transform the tech recruitment process. Its advanced techniques and easily-explainable features deliver a taxonomy that provides valuable insights for recruiters and candidates, and it creates automated sub-products that make the tech workforce ecosystem more efficient and effective.
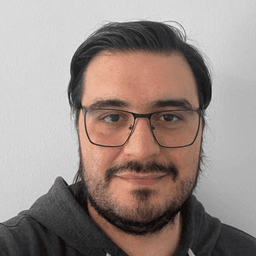